Engineering best practices for Machine Learning
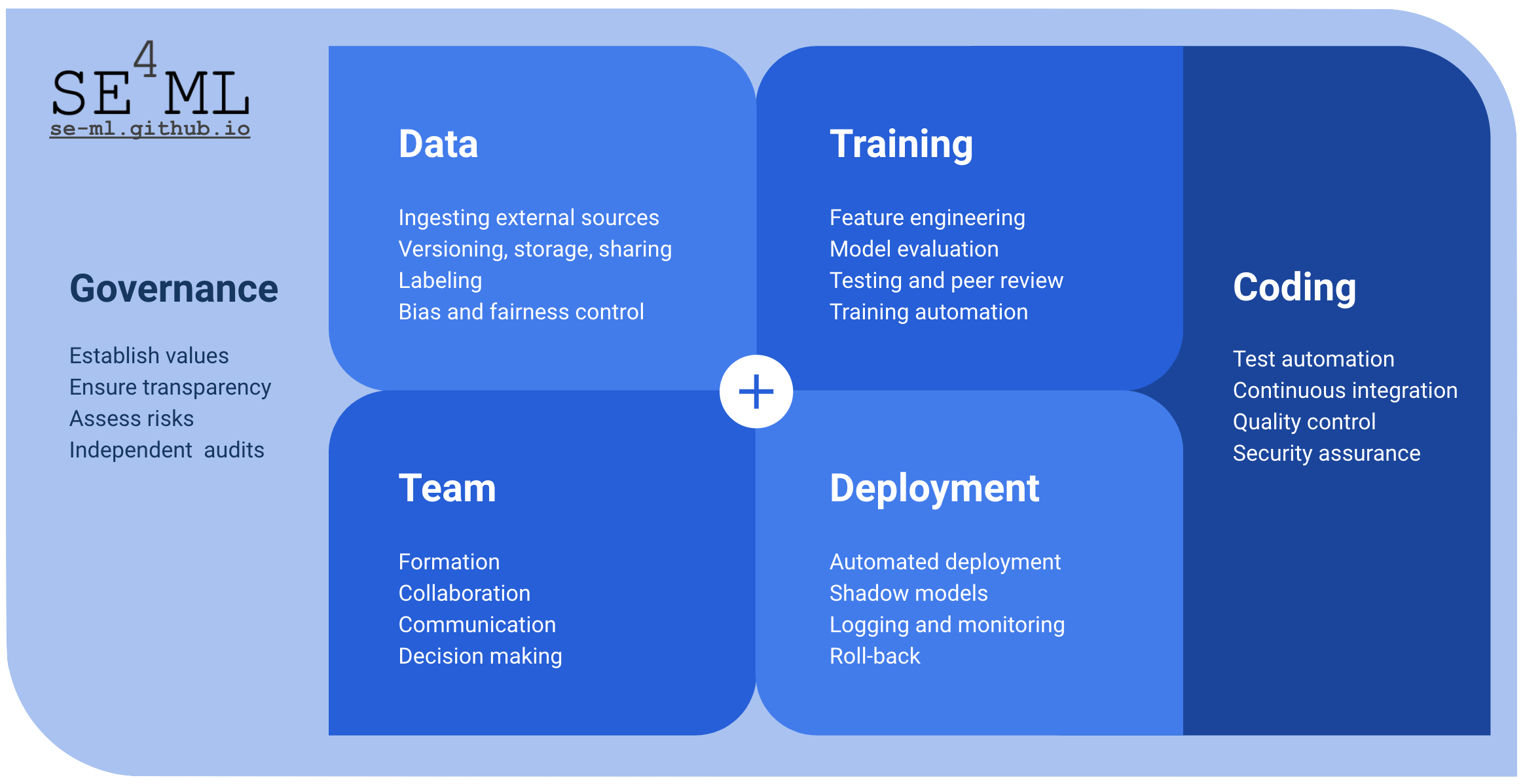
The list below gathers a set of engineering best practices for developing software systems with machine learning (ML) components.
These practices were identified by engaging with ML engineering teams and reviewing relevant academic and grey literature. We are continuously running a global survey among ML engineering teams to measure the adoption of these practices.
The various practices are grouped into 6 categories, as illustrated in the diagram above, and listed below.
The practices are labeled with their difficulty, their effects, and the requirements for trustworthy ML they help to satisfy.
Data
"Whoever owns the data pipeline will own the production pipeline for machine learning." --Chip Huyen- Use Sanity Checks for All External Data Sources
- Check that Input Data is Complete, Balanced and Well Distributed
- Test for Social Bias in Training Data
- Write Reusable Scripts for Data Cleaning and Merging
- Ensure Data Labelling is Performed in a Strictly Controlled Process
- Prevent Discriminatory Data Attributes Used As Model Features
- Use Privacy-Preserving Machine Learning Techniques
- Make Data Sets Available on Shared Infrastructure (private or public)
Training
"No amount of experimentation can ever prove me right; a single experiment can prove me wrong." --Albert Einstein- Share a Clearly Defined Training Objective within the Team
- Capture the Training Objective in a Metric that is Easy to Measure and Understand
- Test all Feature Extraction Code
- Assign an Owner to Each Feature and Document its Rationale
- Actively Remove or Archive Features That are Not Used
- Employ Interpretable Models When Possible
- Peer Review Training Scripts
- Enable Parallel Training Experiments
- Automate Feature Generation and Selection
- Automate Hyper-Parameter Optimisation
- Automate Configuration of Algorithms or Model Structure
- Continuously Measure Model Quality and Performance
- Assess and Manage Subgroup Bias
- Use Versioning for Data, Model, Configurations and Training Scripts
- Share Status and Outcomes of Experiments Within the Team
- Use The Most Efficient Models
Coding
"You can’t be an AI expert these days and not have some grounding in software engineering." --Grady Booch- Run Automated Regression Tests
- Use Continuous Integration
- Use Static Analysis to Check Code Quality
- Assure Application Security
Deployment
“If your model isn’t deployed into production, does it really exist?” --Anonymous- Automate Model Deployment
- Enable Shadow Deployment
- Continuously Monitor the Behaviour of Deployed Models
- Perform Checks to Detect Skew between Models
- Enable Automatic Roll Backs for Production Models
- Log Production Predictions with the Model's Version and Input Data
- Provide Audit Trails
Team
"If you want to go fast, go alone; but if you want to go far, go together." -- African proverb, allegedly- Use A Collaborative Development Platform
- Work Against a Shared Backlog
- Communicate, Align, and Collaborate With Others
- Decide Trade-Offs through Defined Team Process